Next Generation Super Computer Project
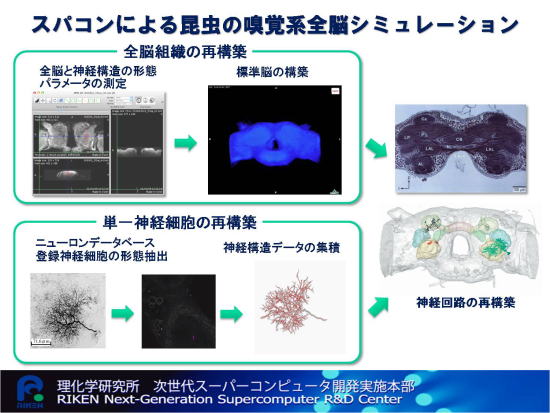
図1.標準脳へのニューロンのマッピング
神経細胞(ニューロン)は情報をスパイク列の形で表現して次に伝える能力を持ち、活動に応じて入出力を変化させるために、知能の礎となっているとされています。系統発生的にヒドラ以上のほぼすべての動物にニューロンは存在し、種によらずその性質はほぼ共通しています。一方、神経回路の規模においては、人間の脳のニューロン数は1011-12個、昆虫は105-6個、線虫では302と種によりかなり差があります。 昆虫は蜜をもつ花を匂いで識別し、その匂いを学習します。また、視覚や嗅覚といった複数感覚情報の統合を脳で行うことが確認されており、わたしたちが知能と定義する活動の多くを昆虫は行っています。これは個体レベルの知能を調べようとする場合、昆虫はその起源に近い重要な位置にあることを示しています。このような立場から、わたしたちは自然が進化で創り上げた知能を理解するために、昆虫の脳、特に雄カイコガの匂い源探索行動をモデルとして、その全過程をシミュレーションすることを目標にしています。わたしたちはカイコガの脳の分析を遺伝子レベル、単一ニューロン、神経回路、行動レベルとさまざまな階層から、分子遺伝学、電気生理学、イメージング、免疫組織学、行動学など多様なアプローチから行っており、多くのデータの蓄積があります。なかでも脳を作るニューロンに関しては、1600個以上から詳細な3次元構造や神経活動を計測しデータベース化しています。このデータベースは個々のニューロンの情報を登録したものとしては世界最大クラスです。これらのデータから雄カイコガの脳内で匂い情報が処理される経路は大域的にはわかっています。 昆虫はニューロン数が少ない割に、高度な知的行動が取れるわけですから、一つのニューロンが大きな役割を果たしていると推測されます。従って、昆虫の大規模神経回路のシミュレーションを行うにあたって、わたしたちは一つのニューロンをマルチコンパートメントモデルを用いて詳しくシミュレーションしています(図2. 動画1)。ニューロンは細長い樹状突起をのばした構造なので、共焦点顕微鏡画像から3次元的に撮影したニューロン形態をシリンダーのつながりとして記述します。等価回路的には一つのシリンダーは細胞外への抵抗、起電力・容量をもち、別のシリンダーとも抵抗でつながっています。入力部は化学シナプスで、神経伝達物質の到着をうけて、5-20ms程度のシナプス後電位がおこります。これがシリンダー中を伝わり、電位依存性Na+チャネルのポジティブフィードバックと電位依存性K+チャネルによる抑制により1-2msの幅をもつスパイク発火を引き起こします(図2C, 動画1)。
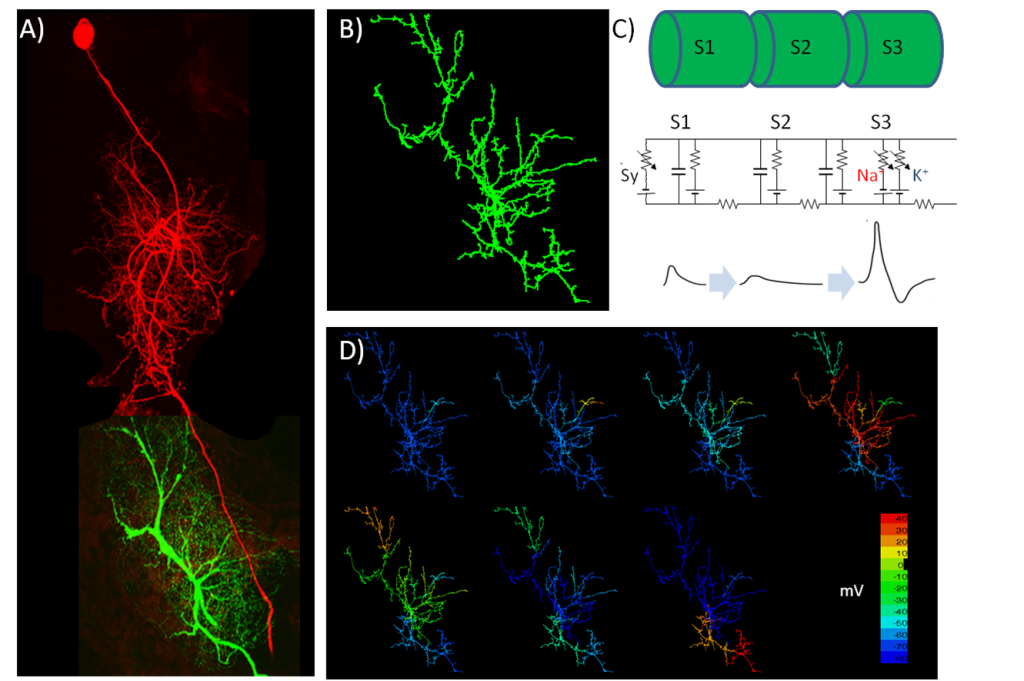
図2.単一ニューロンのマルチコンパートメントモデルによる活動電位の伝播のシミュレーション A:カイコガGⅡ下降性神経(赤)とCv1運動神経(緑)の二重染色像. B:形態抽出されたCv1運動神経. C: マルチコンパートメントモデルと等価回路. D: Cv1運動神経の単一ニューロンのシミュレーション
動画1.単一ニューロンのマルチコンパートメントモデルによる活動電位の伝播のシミュレーション
このように構成されたニューロンを脳内座標にマップして、結合させることで、神経回路シミュレーションが実現できます(図3, 動画2)。 今説明した匂い認識から匂い源探索パターンの生成までには、概算で一万個の脳内のニューロンが関与しています。一つのニューロンを100コンパートメント程度に分けてシミュレーションした場合、ペタフロップスの性能だとリアルタイムでこれらの全体の計算をする能力があると見積もれます。感覚入力から行動出力に至る神経回路のリアルタイムなシミュレーションを行うことができれば、神経回路の一部の入力や出力を計算により置換して脳神経系の情報処理をエンハンスしたり、利用できる可能性が大きく拓けます。また、損傷した脳に対して最小の改変で情報処理機能を回復させるようなニューロリハビリテーションなどの医療につながる設計論を生み出すことが期待されます。
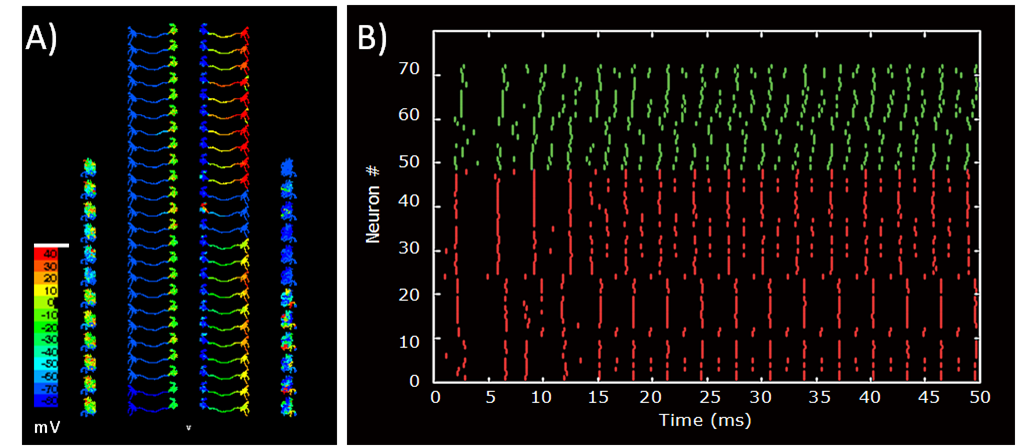
図3.マルチコンパートメントモデルによるカイコガ前運動中枢の神経回路シミュレーション A: 局所介在神経(外側)と両側性神経(内側)の膜電位の空間分布例 B: 局所介在神経(緑)両側性神経(赤)のスパイク発火時刻の分布
動画2.マルチコンパートメントモデルによるカイコガ前運動中枢の神経回路シミュレーション
Nerve cells (neurons) are the building blocks necessary for the emergence of intelligence as they process internal and external information, generally encoded in sequences of spikes and form networks in which such information is relayed between them. Input and output parameters of neurons such as gain can change dynamically depending on prior activity history. Neurons are present in all metazoan animals from simple coelenterates like Hydra and have the same general properties across the animal kingdom. Though the basic building blocks are so similar, there is a big difference in the size of nervous systems, human brains harbor 1011-12 neurons, insects have 105-6 brain neurons, and the somatic nervous system of the nematode Caenorhabditis elegans contains just 302 neurons. Insects are sufficiently complex to display many sophisticated behaviors, such as learning floral odors and colors. Multimodal information is integrated in the insect brain which can generate a wide variety of complex and plastic behaviors, making insect brains a highly suitable system to investigate the emergence of intelligence. Our approach to understanding such an intelligent system shaped by millions of years of evolution is to attempt to recreate it through simulation. This insect whole brain simulation has to encompass information processing from sensory input to behavioral output. We currently focus on the odor-source localization behavior of silkmoth (Bombyx mori) males as a convenient model system. We are analyzing the brain of the silkmoth accumulating numerous data obtained with a large grid of methods including molecular genetics, electrophysiology, imaging, immunohistochemistry, and behavioral experiments. Our methods cover the full scale of observation levels from the molecular and cellular levels to the network and behavior levels. These multi-scale data are integrated in our database, which, for example, contains data on >1600 neurons individually identified in terms of 3D-morphology and physiology, currently making it the largest single-species individual neuron database worldwide. An important insight from these data was the understanding of global and regional connectivity in the olfactory system in the brain of the silkmoth. Since insects can exhibit highly complex behaviors despite having a relatively small number of neurons, it is likely that the relative contribution of each individual neuron is more important than for instance in mammals. In our large-scale simulation of insect neural circuits, we are therefore performing a detailed simulation of each individual neuron using multicompartment models (Fig. 2, Movie 1). The 3D-morphology of neurons is acquired by confocal microscopy and can then be approximated by sets of connected cylinders. A cylinder per se has a simple equivalent circuit consisting of voltage and impedance to the outside of the cylinder (membrane resistance and capacitance). Cylinders are connected by resistors that correspond to axial resistance in neurites. Inputs are described as chemical synapses having postsynaptic potentials of 5-20ms duration upon the simulated activation by neurotransmitter. The synaptic activation can induce spikes of 1-2ms width that are modeled by the conventional sodium and potassium currents (Fig. 2C, Movie 1).
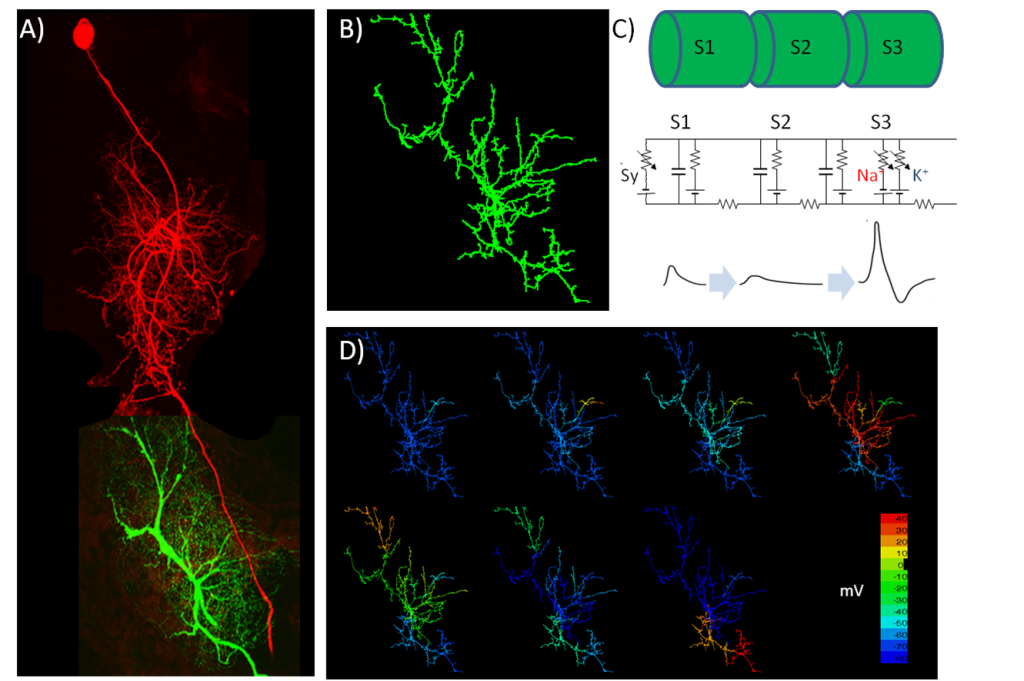
Fig. 1: Simulation with a multicompartment model generated by extraction of neuronal morphology. A) Double-label image of a GII descending neuron and the Cv1 motor neuron B) Extracted morphology of the Cv1 motor neuron C) Multicompartment model and equivalent circuit D) Single neuron simulation of the Cv1 motor neuron
Movie 1:Simulation with a multicompartment model generated by extraction of neuronal morphology.
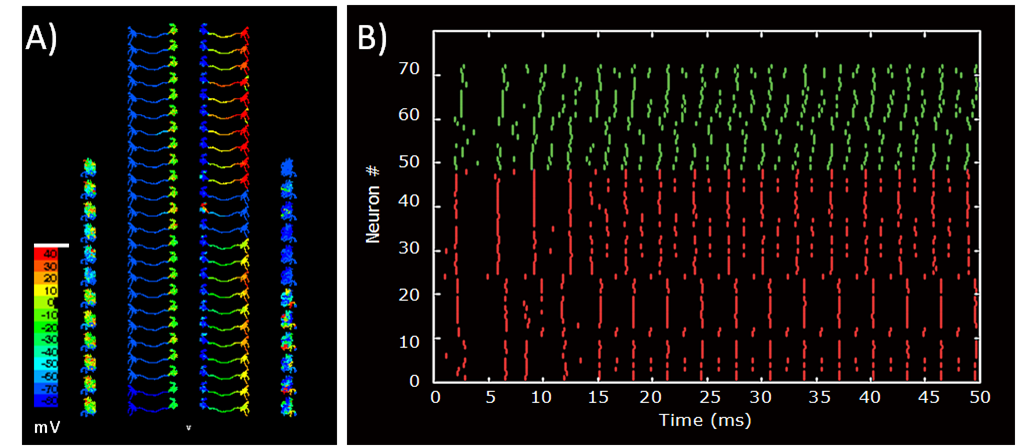
Fig. 3: Neural circuit simulation of the premotor center in the silkmoth brain. A) An example of the spatial distribution of the membrane potentials of local interneurons (outer side) and bilateral neurons (inner side) B) Raster plot of spike events of local interneurons (green) and bilateral neurons (red)
Movie 2:Neural circuit simulation of the premotor center in the silkmoth brain.